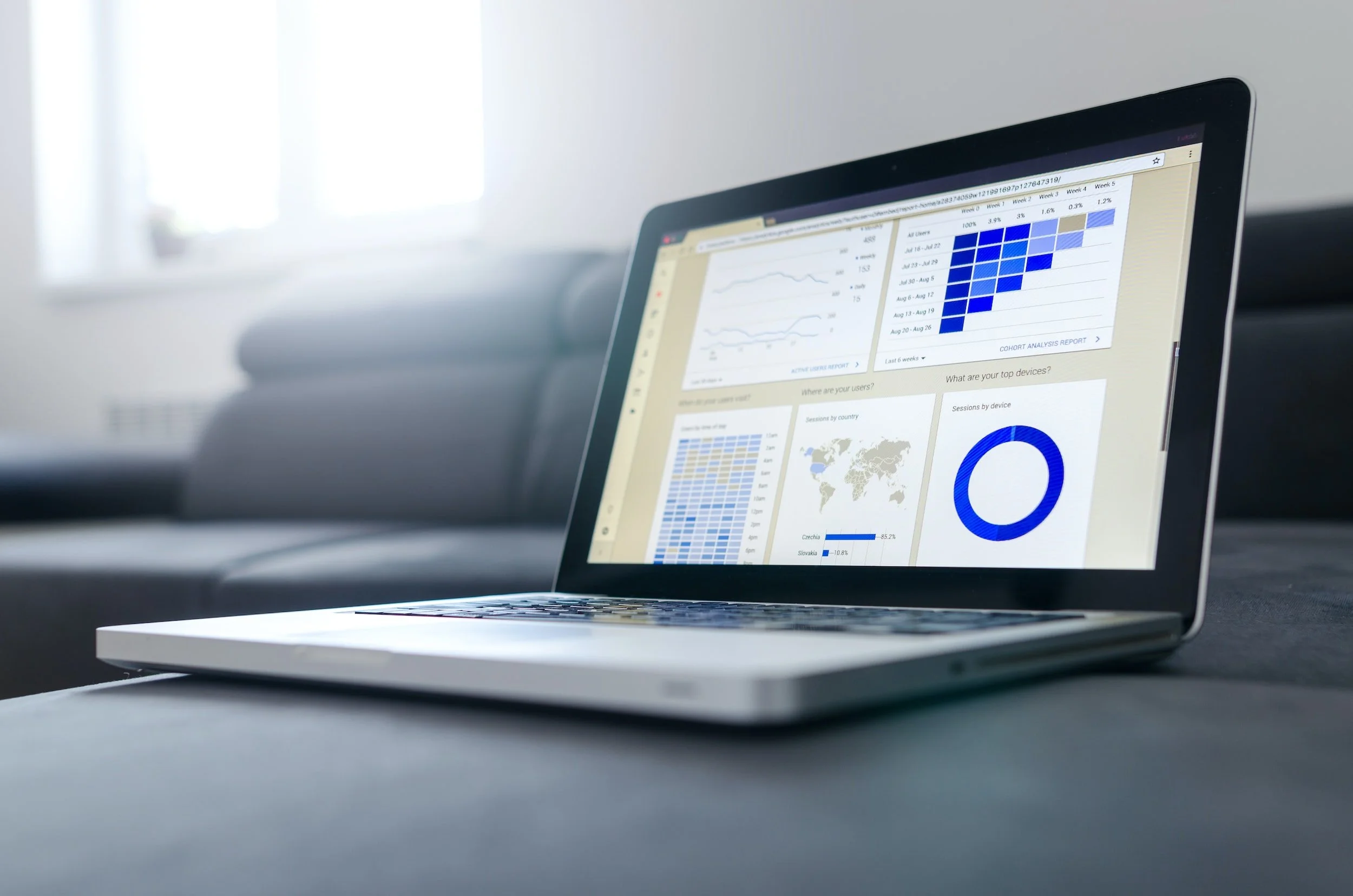
Predicting work absence using secondary data analysis
This study seeks to identify predictive indicators of work absence and develop a model which can accurately predict prolonged work absence in individuals with MSK conditions
Across all working ages a small proportion of people who go on to long-term absence make up the majority of the costs associated with absence from the workplace. However, and particularly for those with MSK pain, there is currently no way of predicting which employees will return to work quickly without additional vocational advice and support, which employees will require this support and what levels of support are most appropriate. Consequently there is no way of ensuring the right individuals are directed towards the right services to support their occupational health needs.
There is a growing evidence base around stratified care approaches to delivering healthcare, but this approach has not yet been developed in occupational healthcare to test whether stratified care according to prognosis (estimated risk of poor outcome- defined as duration of work absence) can be successful in reducing this work absence.
Aims
The aim of this study is to test prognostic indicators of work absence and develop a prognostic model to better identify individuals at risk of prolonged work absence.
This study seeks an answer to the following research question:
Can a prognostic model for prolonged work absence, developed from secondary analysis of cohort studies and clinical trials included in the data resource, accurately predict prolonged work absence in individuals with MSK conditions?
What will this research involve?
Data from randomised controlled trials held at Keele will be pooled allowing variables that are common between studies to be compared.
Variables not collected in studies are similar to those “missing by design” and we would propose using multiple imputation under the assumption that data are missing at random conditional on variables available in all studies.
Secondary analysis of the data resource will be undertaken to test predictors of absence in primary care populations and develop a prognostic model for predicting prolonged work absence.
What has the study found so far?
Publications
Archer, L, Pear, G, Snell, KIE. et al. Musculoskeletal Health and Work: Development and Internal-External Cross-Validation of a Model to Predict Risk of Work Absence and Presenteeism in People Seeking Primary Healthcare. Journal of Occupational Rehabilitation (2024). DOI:10.1007/S10926-024-10223-W
Study team
Chief investigator
Professor Gwenllian Wynne-Jones (Keele University)
Co-investigators
Professor George Peat (Keele University)
Ms Lucinda Archer (Keele University)
Dr Kym Snell (Keele University)
Associated research staff
Professor Danielle van der Windt (Keele University)
Professor Jonathan Hill (Keele University)
Professor Kate Dunn (Keele University)
Professor Nadine Foster (Keele University)
Centre institutions
Further information
If you have any queries about this research please contact g.wynne-jones@keele.ac.uk